Mastering Data Collection in Machine Learning: A Comprehensive Guide
Mastering Data Collection in Machine Learning: A Comprehensive Guide
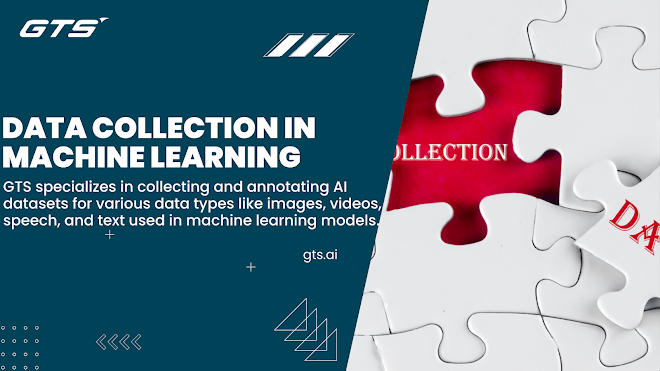
Introduction:
In the field of artificial intelligence, data is the lifeblood that fuels innovation and drives progress. As an integral component of machine learning algorithms, the quality and quantity of data directly impacts the efficacy of AI models. In this comprehensive guide, we shed light on the complexities of Data Collection In Machine Learning, exploring the importance, methods, challenges, and best practices for optimizing this critical process.
Why data collection matters:
Data collection serves as the foundation on which machine learning models are built. It involves collecting relevant information from various sources to train the algorithm effectively. The importance of careful data collection cannot be underestimated, as it directly impacts the accuracy, reliability, and performance of AI systems in various applications.
Data Collection Methods:
- Web Scraping: Leveraging web scraping tools and techniques to extract data from websites, forums, and social media platforms.
- Sensor data acquisition: Collecting data from sensors embedded in IoT devices, wearable devices, and industrial equipment.
- Surveys and Questionnaires: Designing structured surveys and questionnaires to collect specific information from target demographics.
- Public Datasets: Access publicly available datasets from repositories like Kaggle, UCI Machine Learning Repository, and Google Dataset Search.
- Data labeling: Engaging human annotators to label and annotate raw data, facilitating supervised learning tasks.
Challenges in Data Collection:
- Data Quality: Ensuring the accuracy, completeness, and consistency of the data collected.
- Data Privacy: Adhering to ethical standards and regulatory requirements to protect sensitive information.
- Bias and fairness: Reducing implicit biases in data collection that can lead to distorted AI models and discriminatory outcomes.
- Scalability: Managing the scalability of data collection processes to accommodate growing datasets and growing requirements.
- Resource constraints: Dealing with budget, time, and manpower limitations for comprehensive data collection initiatives.
Best Practices for Effective Data Collection:

- Define clear objectives: Establish clear goals and requirements for data collection to guide the process effectively.
- Diversifying data sources: Incorporating diverse sources and types of data to increase the robustness and generalization capabilities of AI models.
- Quality Assurance: Implementing rigorous quality assurance measures to validate the integrity and reliability of the data collected.
- Data Governance: Establishing a strong data governance framework to ensure compliance with regulations and ethical standards.
- Continuous improvement: Iteratively refining data collection strategies based on feedback, insights, and evolving needs to optimize performance.
Conclusion:
In the dynamic landscape of artificial intelligence, mastering the art of data collection is paramount to unlocking the full potential of machine learning algorithms. By adopting systematic methods, overcoming challenges, and adopting best practices, organizations can harness the power of data to drive innovation, gain competitive advantage, and provide transformative solutions across various domains.
Through careful data collection, Globose Technology Solutions remains at the forefront of AI innovation, enabling clients to harness the power of data-driven insights for sustainable growth and success.
Comments
Post a Comment